Update: AI-first Visual Editing
A day after the release of the gpt-image-1
API, we took it for a spin and integrated it into CreativeEditor SDK. Users can now generate images, create variants and use the canvas to compose visual prompts with our design editor. See it in action:
Introduction
The release of OpenAI's gpt-image-1
model signals a pivotal shift in the creative developer landscape—one that moves beyond static, one-shot image generation and toward a more dynamic, multimodal interaction model. Until recently, most image APIs followed a predictable pattern: submit a prompt, receive a finished image. The process was useful, but flat. What’s changing now is not just image quality or style fidelity, but the shape of the workflow itself. With gpt-image-1
, built on the GPT-4o foundation, developers can start designing creative tools that feel conversational and iterative. This evolution invites a new kind of interface where prompting, tweaking, and refining happen inside the canvas, not outside of it.
For teams building creative editing experience into their app, this moment coincides with the release of IMG.LY’s AI Editor SDK, a powerful, fully integrated toolkit designed for generative workflows. The SDK is already equipped to support interactive image generation, contextual editing, and multimodal inputs, and you can try it today through this live demo.
This guide is a comprehensive introduction to the gpt-image-1
API, but it also goes further. It’s not just about wiring up an endpoint, it’s about rethinking what image generation means in a user-centric product.
From prompt handling to interactive iteration, we’ll walk through how to design creative cycles, not just outputs. This guide explores how to make that shift, how to go from generating images to integrating gpt-image-1
into real creative cycles, where AI becomes a tool that bends to user intent, not the other way around.
Overview of gpt-image-1
OpenAI's gpt-image-1
model, released in April 2025, is the latest evolution in the company’s generative image lineup and marks a turning point in how developers approach visual creation inside applications. Built on the same multimodal foundation as GPT-4o, this model allows applications to move beyond one-shot static generation and instead build toward more conversational, iterative image workflows.
Model Architecture and Capabilities
gpt-image-1
is rooted in GPT-4o's ability to understand and generate across modalities. It is designed to produce high-resolution images—up to 4096×4096 pixels—based on natural language prompts. The model handles complex scenes with more fidelity than previous iterations and provides improved consistency in how it interprets detailed descriptions. This is particularly relevant for tools that need reliability when turning prompt inputs into design elements.
Parameter Control
Developers working with gpt-image-1
have access to a streamlined set of parameters, here is a subset of the most important ones:
prompt
: The primary text input describing the desired image.size
: Choose between "1024x1024", "1024x1536" (portrait), "1536x1024" (landscape), or "auto" (default, based on prompt).n
: Number of images to generate (default is 1).response_format
: Always returnsb64_json
. URL outputs are not supported.
Unlike DALL·E 3, gpt-image-1
does not accept style
modifiers or quality
settings. It is designed for straightforward, high-fidelity image creation driven purely by the text prompt and size selection.
Full documentation of these options is available via OpenAI's official guide.
Style and Use Case Alignment
By supporting a wide range of stylistic templates, gpt-image-1
positions itself as a flexible backend for everything from marketing collateral to storyboarding tools. The output can be tailored to suit technical illustrations, concept art, or even photorealistic renderings, allowing developers to map visual outputs more directly to brand or product requirements.
Limitations and Future Direction
As of April 2025, gpt-image-1
supports only one image per request and does not offer fine-grained image editing or inpainting. However, its tight coupling with GPT-4o suggests that future iterations may embrace persistent context, conversational refinement, or even integrated image-plus-text exchanges within the same session. For developers building editors or multimodal workflows, the current model lays a strong foundation for these future capabilities.
API Setup and Usage
2.1 Get Access
To start using gpt-image-1
, developers must first register for access via the OpenAI platform at platform.openai.com. Access requires an API key, which is tied to your OpenAI account and associated usage limits based on your billing tier. Be sure to confirm that your account is approved for image generation, as availability may differ by region and subscription level. Once authenticated, keys can be created in your dashboard and stored securely in your server or development environment.
2.2 First Image Generation (Node.js Example)
The image generation API for gpt-image-1
can be used directly via OpenAI’s official Node.js client. Below is a complete example showing how to send a prompt and receive an image URL in response:
import OpenAI from "openai";
import fs from "fs";
const openai = new OpenAI({
apiKey: process.env.OPENAI_API_KEY, // make sure this is securely set
});
async function generateImage() {
try {
const prompt = `
A studio ghibli style illustration of a cyberpunk girl holding a butterfly on her finger.
`;
const result = await openai.images.generate({
model: "gpt-image-1",
prompt,
size: "1024x1024", // or "1024x1536", "1536x1024", or "auto"
});
const image_base64 = result.data[0].b64_json;
const image_bytes = Buffer.from(image_base64, "base64");
fs.writeFileSync("butterfly.png", image_bytes);
console.log("Image saved as butterfly.png");
} catch (err) {
console.error("Error generating image:", err);
}
}
generateImage();
Remember that all outputs from gpt-image-1
are delivered as base64-encoded JSON. Developers should decode this data for display, storage, or further processing within their applications. For complete parameter options and examples, consult the OpenAI Images API guide.
Integrating with CE.SDK
Embedding gpt-image-1
into a creative editor like CE.SDK is about more than just piping an image into a canvas. It reshapes how users interact with content creation, bridging manual design work and AI-driven generation within the same editing environment. Rather than operating as a standalone prompt generator, gpt-image-1
becomes a continuous creative partner inside your editor. For in in-depth technical guide on how to integrate gpt-image-1
stay tuned for our upcoming tutorial, sign up to our newsletter to be notified when it goes live.
Embedding Image Generation in a Creative Editing Workflow
The natural entry point for gpt-image-1
inside CE.SDK is through a dual-mode experience: offering users the option to start either from scratch or from existing context. In "from scratch" mode, a user might open a blank scene and initiate an image generation by writing a prompt for example, "Create a vibrant festival scene at sunset." The result appears directly on the canvas, immediately editable like any other design element.
Where gpt-image-1
shows its real potential is in "in-context editing." Here, users interact with existing content—a background, a product shot, or a decorative element and trigger AI enhancements based on that visual context. A user might select an image of a bird, as in the example below and ask for variants, initiate a background swap, or request a change like adding more birds in a conversational interface embedded in the editor. Because CE.SDK treats generated images as first-class canvas elements, context such as positioning, layering, and cropping is preserved throughout the process.
Let’s see what this might look like in practice. We positioned an image of a single bird on our canvas, opening the AI context menu we can now manipulate that image in place using the OpenAI API:
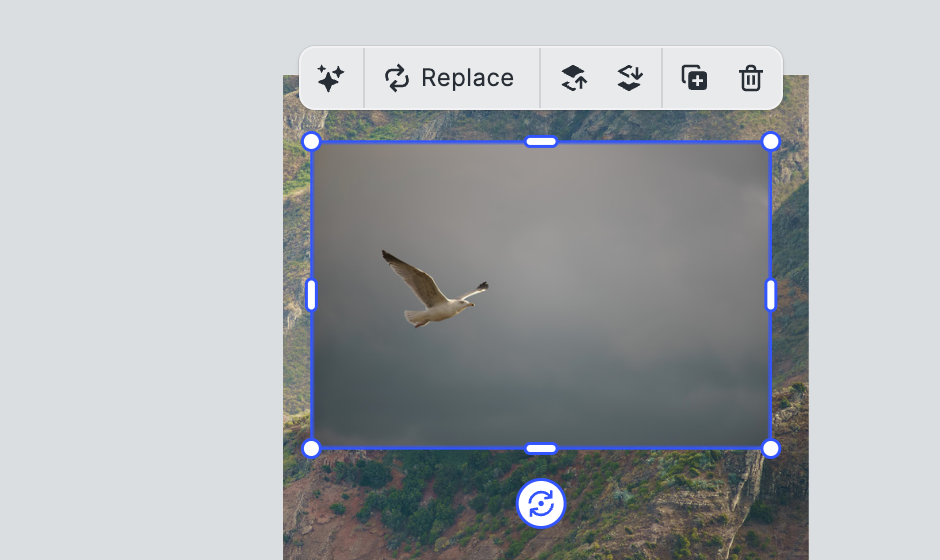
We edit the image and prompt the API to add more birds:
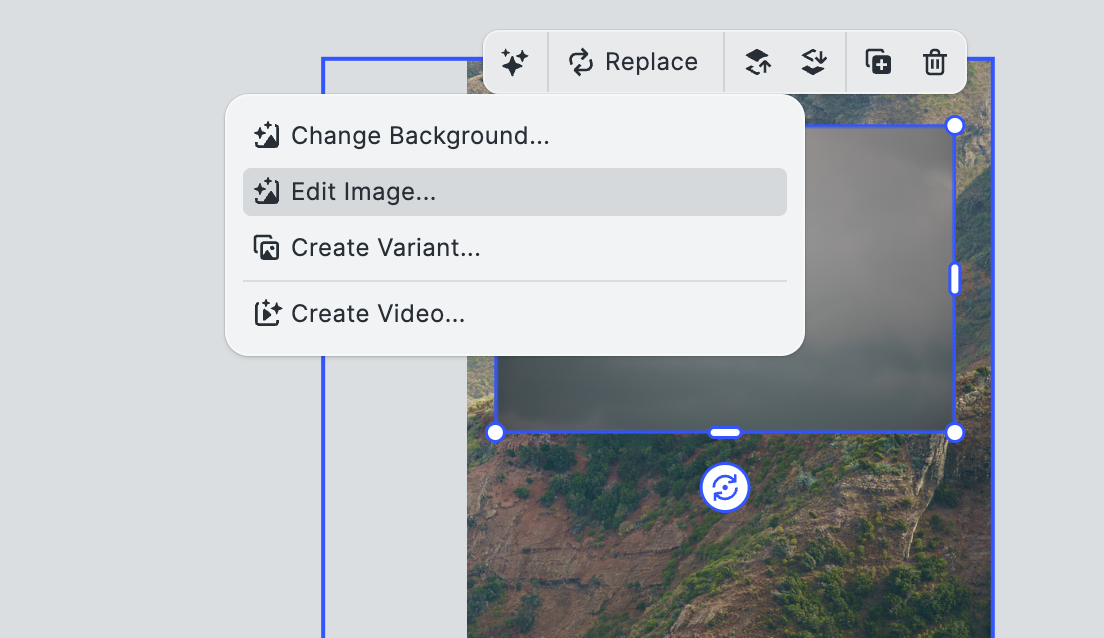
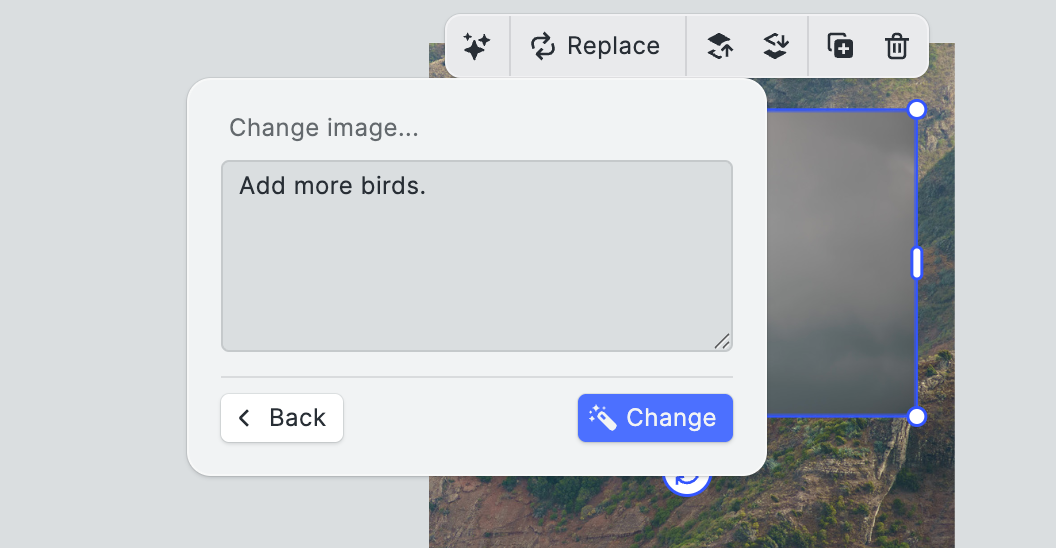
We see that the model correctly identified the type of bird in the picture (seagull) and filled it in with a swarm of flying seagulls.
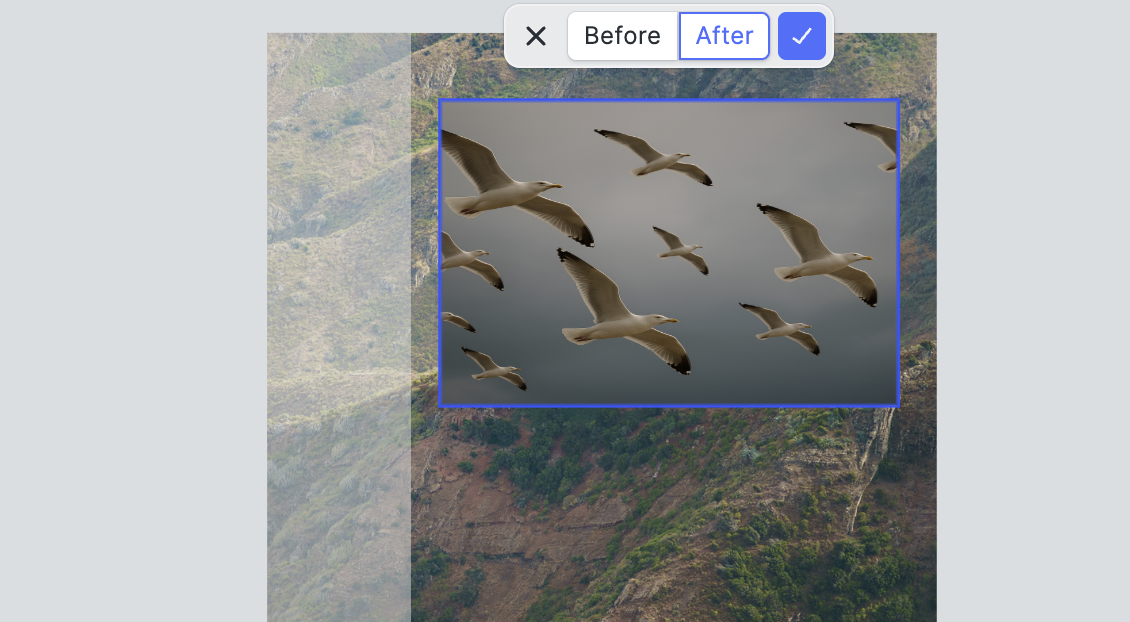
We can now continue to work with the image, overlaying filters, changing the texture, cropping etc.
Switching Between Manual Edits and AI-Powered Enhancements
A critical design principle when integrating gpt-image-1
is giving users freedom to toggle between manual edits and AI suggestions. Manual edits should always remain possible after generation, e.g. cropping, masking, compositing while users can also seamlessly prompt gpt-image-1
for additional changes without losing prior work. Think of variant generation as a branch: a user picks a generated image and creates "forks" by asking for alternate styles, different lighting, or new thematic elements.
In this setup, the generated image serves as a stable node in the creative graph, while edits and regenerations can attach contextually. This workflow minimizes user frustration by avoiding the "start over" penalty typical of isolated generation APIs. It also opens up more complex creative behaviors, like blending user-drawn sketches with AI-augmented refinements, or iteratively developing an asset library around a consistent visual theme.
An upcoming in-depth tutorial will walk through implementing this multimodal workflow step-by-step, but the key takeaway is that gpt-image-1
shines brightest when it is embedded into a creative loop—not treated as a black-box generator, but as an interactive, iterative design companion.
Prompt Engineering Tips
One of the most overlooked but critical factors in successful image generation is prompt design. With gpt-image-1
, prompt engineering isn't just about describing an image—it's about steering the model toward intent, tone, composition, and usability. Because the model is capable of rendering complex scenes and a wide range of styles, thoughtful phrasing and contextual hints can dramatically affect the outcome.
Writing for Visual Intent
Start by clarifying what the image is supposed to communicate. Are you looking for atmosphere, action, product detail, or narrative clarity? A prompt like "a city skyline at night" is a starting point, but it leaves too much to chance. Adding elements like "view from a rooftop bar, with glowing signage and overcast haze" gives the model anchors for both composition and mood.
Leveraging Artistic Language
You can further refine outputs by referencing mediums or artistic schools. Prompts that include terms like "in watercolor style," "oil painting," "80s anime aesthetic," or "studio photography" help the model lock onto a particular visual identity. These cues not only improve stylistic fidelity but also align the output with specific brand or genre expectations, which is especially important for products with a defined look and feel.
Creating Consistency in Branded Outputs
When generating a set of related images, such as social media creatives, campaign assets, or UI visuals, consistency becomes more important than variety. To achieve this, structure prompts with repeatable patterns and include brand elements such as color palettes, motifs, or reference characters. While gpt-image-1
doesn't yet support persistent memory across requests, consistency can be enforced by prompting with the same style terms, layout descriptions, and constraints. Teams working within CE.SDK can even pair prompt templates with locked canvas layers to preserve composition between generations.
Ultimately, good prompt engineering is not about verbosity but about clarity and constraint. It's less like writing poetry and more like drafting a product spec. The best prompts are focused, directive, and give the model just enough creative freedom within clear boundaries. However, effective prompting should not burden the user. In practice, the interface should abstract most of the complexity away. Users can be guided toward better outputs through simple UI choices—selecting predefined styles, choosing themes, or adjusting mood settings—while the system dynamically enhances and augments their input behind the scenes. By managing the technical depth invisibly, you enable a creative process that feels intuitive and powerful without ever making prompt engineering the center of the user experience.
Real-World Use Cases
The versatility of gpt-image-1
makes it especially impactful across a variety of industries where visual content creation is either a core product feature or a major operational need. Beyond isolated image generation, the model supports workflows that demand contextual awareness, brand consistency, and iterative refinement, key ingredients for modern digital products.
Web-to-Print
In web-to-print applications, customers expect to customize marketing materials, event invitations, signage, or packaging with minimal friction. By integrating gpt-image-1
, developers can offer template-driven personalization where users simply select a theme or enter a few keywords, and receive ready-to-edit visual assets. Combined with CE.SDK’s layout and editing capabilities, this enables a highly interactive experience where generated backgrounds, graphical elements, or themed illustrations can be dynamically placed into editable templates.
Social Media Marketing and MarTech
Marketing teams rely on high-frequency content creation, often needing visually consistent, campaign-specific assets. gpt-image-1
can assist by automating the generation of background scenes, promotional visuals, and thematic graphics based on campaign briefs. Brands can define style presets aligned with their visual identity, making it easy for marketing teams to produce "on-brand" assets without heavy design overhead. Integrating image generation directly into campaign builders or social scheduling tools amplifies speed without sacrificing quality.
Digital Asset Management (DAM)
Asset libraries often suffer from gaps: missing variants, seasonal versions, or content tailored to different demographics. DAM systems can integrate gpt-image-1
to extend asset catalogs dynamically. Instead of manually commissioning variations, users can generate alternative backgrounds, localize visuals with region-specific elements, or adjust brand visuals for different markets—all from a single master file. With CE.SDK handling structured editing, teams maintain asset consistency while boosting creative flexibility.
E-Commerce
Product visualization remains a huge challenge in e-commerce, especially for smaller retailers. gpt-image-1
can be used to automatically create product lifestyle imagery, context backgrounds, or thematic campaigns without expensive photo shoots. For example, a single shoe photograph can be placed into a generated "urban," "sporty," or "luxury" background, customized according to target audiences. When tightly integrated into e-commerce platforms, this enables faster product launches, A/B tested visuals, and localized campaigns at scale.
E-Learning
Educational platforms can harness gpt-image-1
to generate explanatory diagrams, thematic illustrations, or scene-based visual storytelling assets. Instead of relying solely on static stock imagery, teachers, course designers, or even learners themselves can prompt the generation of custom visuals aligned with the curriculum. When embedded into authoring tools, this approach accelerates content creation and enables more engaging, visually enriched learning experiences tailored to specific topics and age groups.
Cost Optimization
While gpt-image-1
opens up impressive creative possibilities, it also introduces new cost considerations that developers and product teams must plan for carefully. Since image generation typically incurs higher API costs than text-based operations, structuring workflows efficiently becomes critical, especially at scale.
Balancing Price, Quality, and Resolution
The cost of generating an image with gpt-image-1
depends significantly on both the requested resolution and the selected quality setting. Higher resolutions like 4096×4096 produce sharper, more detailed results, but they also consume more compute resources-and therefore cost more. For many use cases, especially for previews, lower resolutions such as 1024×1024 or 2048×2048 strike an excellent balance between visual fidelity and API efficiency. Reserving the highest quality settings for final exports or premium workflows can help manage overall spend without compromising user experience.
Image Reuse and Smart Upscaling
One practical cost-saving approach is to design workflows that encourage image reuse. Instead of regenerating similar images for every small variation, applications can create high-quality master images and allow users to crop, edit, or layer additional design elements dynamically. Integrating smart upscaling techniques-for instance, using specialized image enhancement libraries after initial generation-also allows teams to work with smaller base images without sacrificing end-user quality.
Rate Limits and Batching Strategies
Every call to gpt-image-1
counts toward your usage quota, and OpenAI imposes rate limits depending on account tier. To optimize performance and cost, it's helpful to batch generation requests thoughtfully where possible-for instance, combining multiple prompts into structured queues or allowing users to preview low-res draft versions before finalizing a high-res render. Building this logic into your app’s generation flow not only controls expenses but also improves perceived responsiveness, an important UX factor for creative applications.
By considering cost optimization as an early design constraint rather than a late-stage patch, developers can build scalable, sustainable creative tools powered by gpt-image-1
.
Bonus: Starter Kit Repo
We are currently in the process of integrating the new GPT-4o-powered gpt-image-1
model into CE.SDK. As part of this effort, we are preparing a comprehensive Starter Kit will showcase a complete with CE.SDK integration, real-time prompt input, image generation workflows, and best practices for building an AI-powered creative editor.
Both a public GitHub repository and a live demo will be made available soon. If you want to be notified when the Starter Kit launches, you can subscribe to updates here.
This Starter Kit is designed to help developers move beyond simple image generation into building full creative cycles, where users can generate, edit, refine, and remix visuals seamlessly inside the editor.
FAQs
Choosing to work with gpt-image-1
raises a number of practical and strategic questions. Below, we address the most common topics for teams evaluating the model for integration into creative workflows.
How is gpt-image-1
different from DALL·E 3?
While DALL·E 3 and gpt-image-1
both translate text prompts into images, the underlying architecture and integration paths are different. gpt-image-1
is built on GPT-4o's multimodal framework, making it better suited for future conversational and iterative workflows. It also offers support for a wider range of styles, higher resolutions up to 4096×4096 pixels, and is positioned for deeper integration into dynamic user experiences rather than one-off generation tasks.
Can you fine-tune or train gpt-image-1
?
As of April 2025, OpenAI does not allow fine-tuning of gpt-image-1
. The model is optimized for broad creative use cases out of the box. Developers seeking more control typically customize the user-facing prompt engineering or combine outputs with structured editing tools like CE.SDK to achieve brand or project-specific consistency.
Is offline support available?
Currently, gpt-image-1
requires access to OpenAI's cloud APIs. There is no offline inference mode or local deployment option. Teams requiring strict data residency, offline workflows, or private model hosting should consider hybrid architectures where images are generated securely via backend services and then edited locally using embedded tools like CE.SDK.
What about copyright and licensing?
Images generated by gpt-image-1
can be used commercially according to OpenAI’s usage policies, but developers are encouraged to review the latest terms. Outputs are not directly copyrighted by OpenAI or the user, and responsibility for ensuring compliance with branding, likeness, or content standards typically falls on the developer or platform operator. When deploying generation features to end-users, it is good practice to provide clear terms of use and, if needed, additional moderation or review layers.
By addressing these considerations early, teams can integrate gpt-image-1
more effectively and responsibly into creative products and workflows.
Conclusion
gpt-image-1
offers developers a significant opportunity to rethink what image generation can mean inside creative applications. It is not simply a tool for producing pictures on command, but a foundation for building interactive, iterative design workflows where users stay in control of the creative process. When combined with CE.SDK, it becomes even easier to move from static outputs to living, editable canvases that support real-world design needs. As we continue to integrate GPT-4o capabilities, the next wave of creative tooling will be about more than prompting images-it will be about shaping truly collaborative creative environments. Now is the time to start experimenting, iterating, and reimagining the user experience around this new generation of multimodal AI.